CogniNet++: A Hybrid Deep Learning Framework for EEG-Based Cognitive State Classification Using Spatial-Temporal Attention and Multi-Task Learning
DOI:
https://doi.org/10.70162/smrj/2025/v3/i1/v3i1s07Keywords:
EEG signal analysis, cognitive state classification, deep learning, graph neural networks, attention mechanism, multi-task learningAbstract
Accurate classification of cognitive states such as stress, focus, and mental workload from electroencephalography (EEG) signals is essential for developing real-time, adaptive neurotechnology. Traditional models struggle to generalize due to the high dimensionality and temporal variability of EEG data. This study proposes a novel deep learning architecture, CogniNet++, designed to enhance the accuracy and robustness of EEG-based cognitive state classification. CogniNet++ integrates temporal convolutional layers, graph neural networks (GNNs) for spatial encoding, and Transformer-based self-attention for long-range temporal dependencies. The architecture also includes dual attention modules that dynamically focus on relevant time steps and electrode regions. A multi-task learning framework enables simultaneous classification of three cognitive states. The model is evaluated on the CLARE dataset, which includes 32-channel EEG recordings with labeled stress, focus, and workload conditions. Preprocessing steps include bandpass filtering (1–50 Hz), independent component analysis (ICA), and z-score normalization. CogniNet++ achieves classification accuracies of 86.9% for stress, 84.7% for focus, and 82.1% for workload, outperforming baseline models including CNN, LSTM, EEGNet, and Transformer-only architectures. Macro F1-scores and ROC-AUC metrics also demonstrate superior performance, with all improvements statistically significant (p < 0.05). The proposed model demonstrates state-of-the-art performance in multi-state EEG classification. By effectively capturing spatial-temporal dynamics, CogniNet++ advances the field of cognitive monitoring and offers scalable potential for real-world applications such as education, neurofeedback, and mental health diagnostics
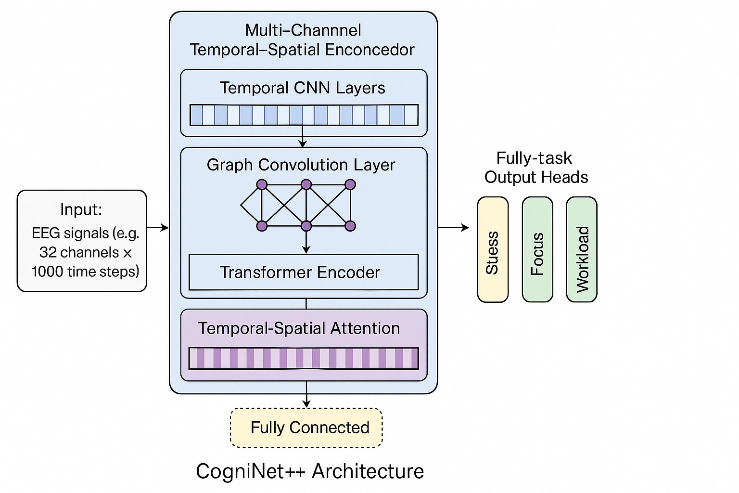
Downloads
Published
Issue
Section
License

This work is licensed under a Creative Commons Attribution-NonCommercial 4.0 International License.
This work is licensed under a Creative Commons Attribution-NonCommercial 4.0 International License. You are free to share and adapt the material, but only for non-commercial purposes. You must give appropriate credit to the author(s).