Lightweight Machine Learning-Based Static Analysis Framework for Real-Time Android Malware Detection
DOI:
https://doi.org/10.70162/smrj/2025/v3/i1/v3i1s06Keywords:
Prescription Toxicity, BiLSTM, Attention Mechanism, Ensemble Learning, Opioid Risk Prediction, InterpretabilityAbstract
The rising incidence of opioid-related prescription toxicity poses a serious public health threat, necessitating intelligent systems that can accurately identify high-risk prescribers and patterns in large-scale clinical datasets. Traditional machine learning models fall short in capturing temporal dependencies and providing actionable interpretability in such settings. This study aims to develop a robust, interpretable, and scalable framework for predicting prescription toxicity using deep learning and ensemble methods. We propose a hybrid model that integrates a Temporal Attention-Based Bidirectional Long Short-Term Memory (BiLSTM) network with a Meta-Ensemble Voting Classifier comprising XGBoost, CatBoost, and LightGBM. The model utilizes sequential patterns from time-ordered prescriptions alongside static features extracted from the publicly available CMS Medicare Part D dataset. Attention mechanisms are incorporated to identify and emphasize temporally significant prescription events, while SHAP-based analysis provides global and local feature interpretability. The proposed model outperformed several baselines including Logistic Regression, SVM, Random Forest, and standalone BiLSTM. It achieved an accuracy of 91.4%, an F1-score of 88.7%, and an AUC-ROC of 0.944, demonstrating superior predictive power and generalization. The confusion matrix indicated a high true positive rate with minimal false positives, and attention heatmaps revealed strong alignment with known high-risk prescription patterns. In conclusion, this research presents a novel and interpretable deep ensemble framework that bridges the gap between sequential modeling and structured data analysis. The model’s performance and transparency position it as a viable tool for deployment in clinical risk assessment, policy evaluation, and real-time opioid toxicity surveillance
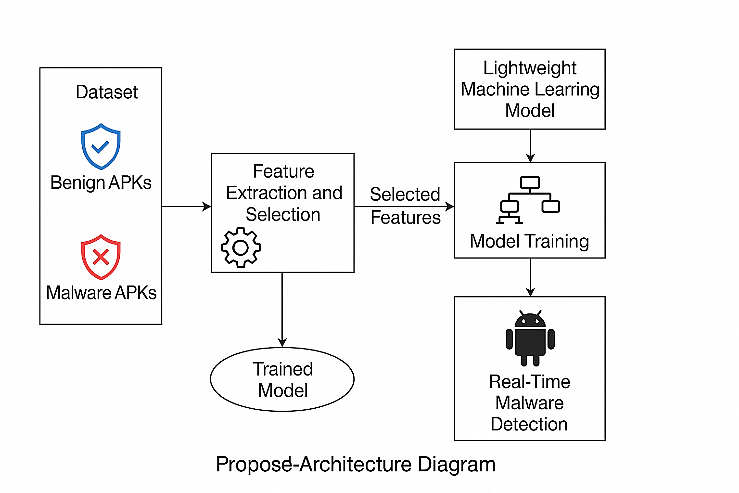
Published
Issue
Section
License

This work is licensed under a Creative Commons Attribution-NonCommercial 4.0 International License.
This work is licensed under a Creative Commons Attribution-NonCommercial 4.0 International License. You are free to share and adapt the material, but only for non-commercial purposes. You must give appropriate credit to the author(s).