Anomaly Detection in Banking Transactions Using Isolation Forest and Temporal Attention-Based LSTM Deep Learning Model
DOI:
https://doi.org/10.70162/smrj/2025/v3/i1/v3i1s02Keywords:
Anomaly Detection, Fraud Detection, LSTM, Isolation Forest, Autoencoder, Bi-LSTM, Meta-Learning, Transaction Analysis, Banking SecurityAbstract
The increasing complexity and volume of digital banking transactions have made traditional rule-based fraud detection systems inadequate due to their high false-positive rates and limited adaptability to evolving fraud patterns. Accurate and interpretable fraud detection in real-time remains a critical challenge in modern financial systems. This study aims to develop a robust and scalable hybrid anomaly detection framework that combines statistical learning, deep sequential modeling, and attention-based mechanisms to improve the detection of fraudulent transactions in banking environments. The proposed model, termed the Multi-View Temporal Attention Model (MV-TAM), integrates multi-view feature encoding (monetary, behavioral, and temporal features), Bi-directional LSTM with attention, and unsupervised anomaly scoring techniques using Isolation Forest and Autoencoders. An end-to-end score fusion strategy is employed, followed by classification via a lightweight meta-learner. Experiments were conducted on the publicly available Kaggle Credit Card Fraud Detection dataset consisting of 284,807 transactions, of which 492 are labeled as fraud. MV-TAM achieved an F1-score of 0.87, AUC-ROC of 0.96, and reduced the False Positive Rate to 0.009, outperforming baselines including standard LSTM, Isolation Forest, and Random Forest classifiers. This research contributes a high-performing and interpretable fraud detection system, implementable in real-time financial applications, and adaptable to imbalanced, high-dimensional transaction environments, addressing both detection accuracy and operational reliability
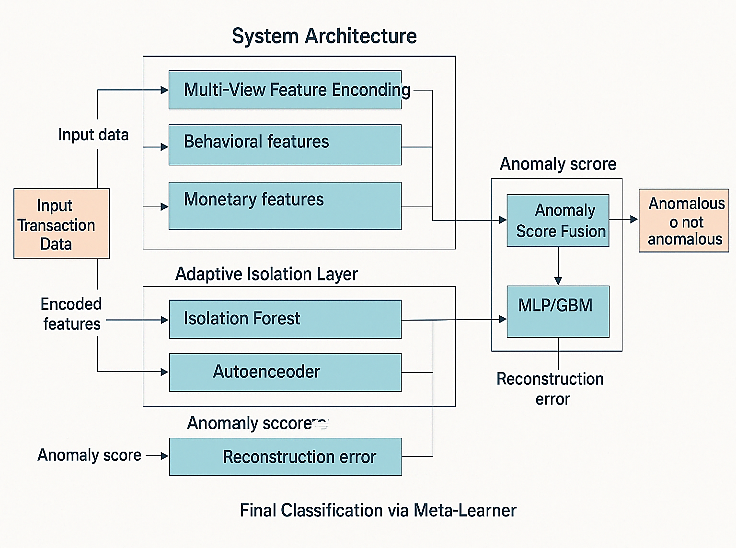
Published
Issue
Section
License

This work is licensed under a Creative Commons Attribution-NonCommercial 4.0 International License.
This work is licensed under a Creative Commons Attribution-NonCommercial 4.0 International License. You are free to share and adapt the material, but only for non-commercial purposes. You must give appropriate credit to the author(s).