Advancing Image Classification and Detection Using CNNs: High Accuracy, Scalability, and Generalization
DOI:
https://doi.org/10.70162/fcr/2024/v2/i1/v2i1s04Keywords:
Artificial Intelligence (AI), Artificial Neural Networks (ANN), YOLO and Faster R-CNNAbstract
The exponential growth of visual data has spurred significant advancements in image classification and detection, with artificial intelligence (AI) playing a pivotal role in achieving high accuracy and scalability. This study presents a CNN-based methodology for image classification and object detection, leveraging supervised learning to classify over 2,000 images of cats and dogs and detect objects within augmented datasets to enhance diversity. The proposed model achieved notable results, with accuracy metrics of 91.32% for cat classification and 90.17% for dog classification, alongside high detection precision. Precision and recall metrics further underscored the model’s effectiveness, yielding F1 scores of 91.31% and 89.93% for cat and dog classifications, respectively. Object detection capabilities were evaluated using models such as YOLO and Faster R-CNN, achieving a mean average precision (mAP) of 89.2% across diverse image datasets. Comparative analyses revealed that the CNN-based approach significantly outperformed traditional regression models, with improvements exceeding 25% in classification accuracy and robust object localization performance. The model’s scalability was demonstrated through consistent results across augmented datasets, achieving an average accuracy of 90.58%. Error analysis highlighted challenges in images with overlapping features, suggesting avenues for improvement through attention mechanisms. This study provides a comprehensive framework for advancing image classification and detection systems across diverse applications, such as medical imaging, autonomous driving, and e-commerce platforms.
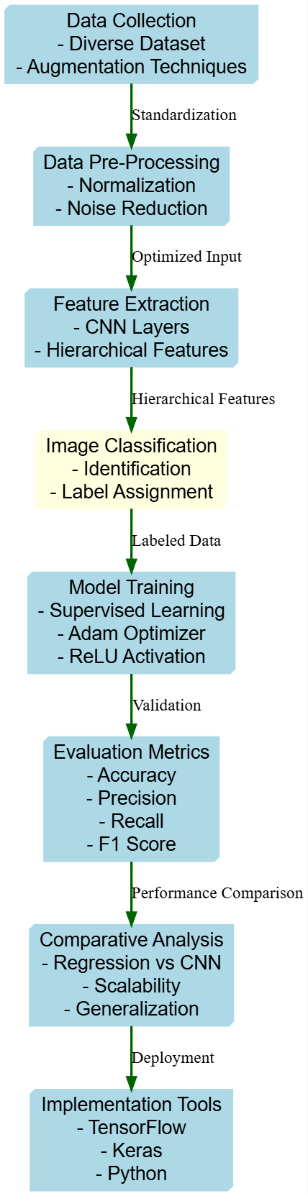
Downloads
Published
Issue
Section
License

This work is licensed under a Creative Commons Attribution 4.0 International License.
Public Licensing Terms
This work is licensed under a Creative Commons Attribution 4.0 International License (CC BY 4.0).
You are free to:
- Share: Copy and redistribute the material in any medium or format.
- Adapt: Remix, transform, and build upon the material for any purpose, even commercially.
Under the following terms:
- Attribution: You must give appropriate credit, provide a link to the license, and indicate if changes were made. You may do so in any reasonable manner, but not in any way that suggests the licensor endorses you or your use.
To view a copy of this license, visit http://creativecommons.org/licenses/by/4.0/.