Transformer-Based Adaptive Examination Systems for Scalable, Accurate, and Personalized Assessments in Education
DOI:
https://doi.org/10.70162/fcr/2024/v2/i1/v2i1s02Keywords:
Adaptive Examination System; Transformer-Based NLP; Question Generation; Response Evaluation; Adaptive Learning; Artificial Intelligence in Education; Semantic Similarity; Personalized Assessments; Natural Language Processing; Educational TechnologyAbstract
This study proposes an adaptive examination system that leverages transformer-based natural language processing (NLP) models to revolutionize educational assessments. Utilizing T5 for question generation and BERT for response evaluation, the system dynamically adjusts question difficulty based on learners' performance, ensuring a personalized and equitable experience. The adaptive framework demonstrated superior performance, achieving evaluation accuracies up to 18% higher than traditional machine learning models and 32% better than rule-based approaches. The system effectively generates diverse questions, spanning cognitive domains from recall to higher-order reasoning, and evaluates responses using semantic similarity, enabling nuanced grading. Experimental results show a balanced distribution of difficulty levels, enhancing student engagement without overwhelming or under-challenging learners. Despite computational overhead and potential biases in pre-trained models, the system addresses scalability challenges through model optimization techniques. This research highlights the transformative potential of AI in education, offering a scalable, efficient, and inclusive solution to modern assessment needs. Future work aims to expand multilingual capabilities and integrate advanced adaptive mechanisms for enhanced personalization.
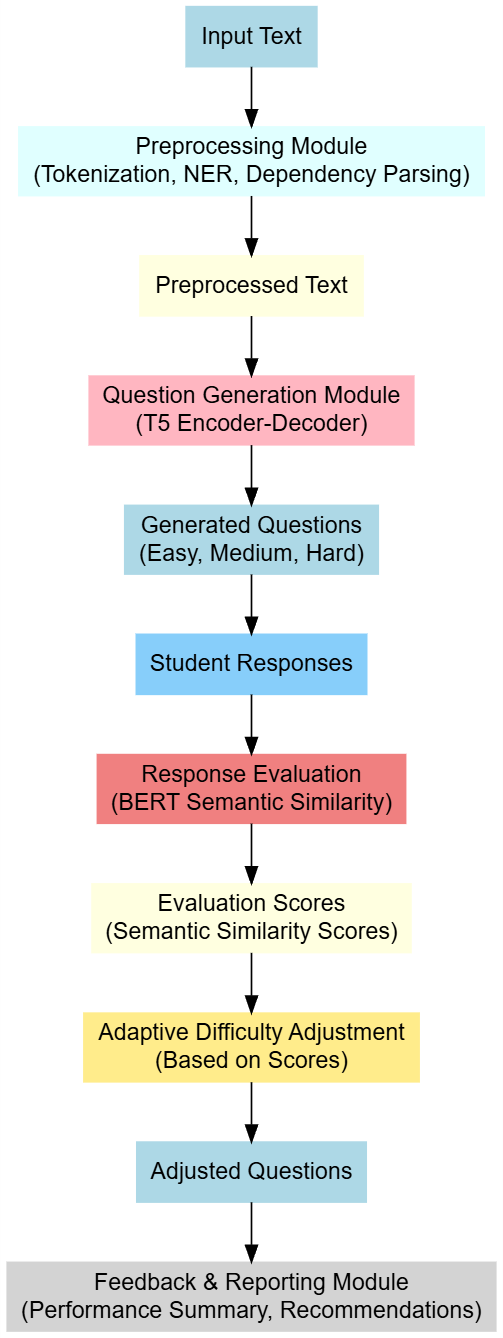
Downloads
Published
Issue
Section
License

This work is licensed under a Creative Commons Attribution 4.0 International License.
Public Licensing Terms
This work is licensed under a Creative Commons Attribution 4.0 International License (CC BY 4.0).
You are free to:
- Share: Copy and redistribute the material in any medium or format.
- Adapt: Remix, transform, and build upon the material for any purpose, even commercially.
Under the following terms:
- Attribution: You must give appropriate credit, provide a link to the license, and indicate if changes were made. You may do so in any reasonable manner, but not in any way that suggests the licensor endorses you or your use.
To view a copy of this license, visit http://creativecommons.org/licenses/by/4.0/.